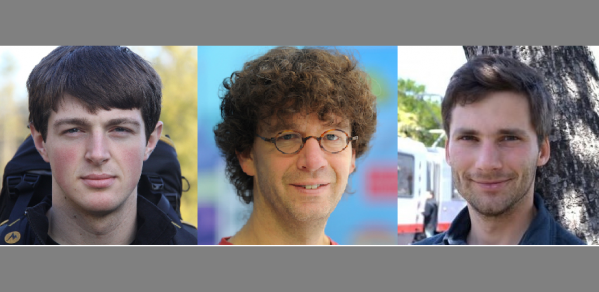
PhD student David Burt has won the Best Paper Award at the International Conference on Machine Learning 2019.
Gaussian process regression is the gold standard for many tasks in terms of quantifying uncertainty about predictions.
Alumnus Mark van der Wilk
In sunny Southern California, the world’s community of AI researchers had one of its biggest annual gatherings, the International Conference on Machine Learning (ICML). David Burt and his team, Carl Edward Rasmussen and Mark van der Wilk won the Best Paper Award their publication 'Rates of Convergence for Sparse Variational Gaussian Process Regression', in which they analysed the computational effort needed to accurately approximate Gaussian process regression.
David is a first year PhD student in the Computational and Biological Learning (CBL) Lab. The research was a collaboration between CBL in the Department of Engineering and Cambridge start-up company Prowler.io.
Gaussian process regression is the gold standard for many tasks in terms of quantifying uncertainty about predictions. The computational cost of most implementations of exact Gaussian process regression, scale cubically with the number of data points, which means they cannot be applied to many of the large datasets that are common nowadays.
Fast approximations were developed, but the trade-off between accuracy and computation for these approximations when applied to large datasets was unknown. The paper addresses this by showing that in a common case, the approximation can be made as accurate as desired, while still scaling to large data much better than the exact method.
While Gaussian Processes still require research to make them more efficient, it is encouraging to now know that the growth of the computational cost is not a fundamental limit to what can be achieved.
Reference:
David R. Burt, Carl E. Rasmussen, Mark van der Wilk Rates of Convergence for Sparse Variational Gaussian Process Regression'