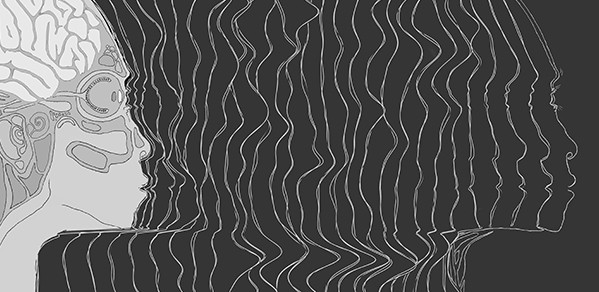
Our brains are highly skilled at learning patterns in the world and making sense of them. The brain continually learns and adapts throughout our lives, and even the neurons supporting learned behaviours, such as the daily walk to work, are constantly changing.
These changes in the neural code bear similarities to how languages change gradually over time, while faithfully communicating common ideas and concepts.
Dr Michael E. Rule
This “representational drift” occurs without any obvious change in behaviour or task performance. Everything seems routine and stable, i.e. you follow the same path to work, make the same plan and take the same steps, but all the while, patterns of neural activity in certain parts of the brain are changing. A new study, published in the journal PNAS, proposes how the brain stays stable despite changes in the neural code.
Cambridge neuroscientists and study co-authors Dr Michael E. Rule and Dr Timothy O'Leary, argue that neurons (the cells that make your brain work) can detect when some of their inputs change, and adjust the strength of influence that one neuron has on another, in order to compensate, thus supporting a form of internal learning.
“These changes in the neural code bear similarities to how languages change gradually over time, while faithfully communicating common ideas and concepts,” says Dr Rule, a Leverhulme Early Career Fellow in the Department of Engineering.
While some parts of the brain are plastic, and change rapidly, other parts show long-term stability. So how do neural circuits talk to each other without continuously having to re-learn the things that they have already learned? Even brain-machine interfaces – which are increasingly being used as assisted living devices for people with cognitive or physical impairments – must contend with “drift”.
The researchers argue that homeostatic processes within single cells can help the brain to ‘watch itself’ as it changes, and that internally-generated signals help stable neural populations ‘learn’ how to track the unstable ones. The researchers made this conjecture based on modelling and on data/observations of living brain activity.
Just how engineers are currently developing machine learning algorithms to track neural representations as they change – automatically – the researchers propose that something similar to these algorithms could also be at work in the brain, emerging from well-known learning rules and homeostatic processes.
“This might explain how plastic and stable neural populations are able to coexist in the brain,” said Dr Rule. “We already know that ‘representational drift’ happens in the hippocampus – the part of the brain that has a major role in learning and memory – and seems to happen in the parietal cortex – the area responsible for sensory perception and integration. What we propose are several specific mechanisms that could help make this plasticity compatible with long-term stability through the brain.”
Dr O’Leary, Associate Professor in the Department of Engineering, said the study emphasises the idea that “drift” may arise from continual learning.
“There is a huge unanswered challenge in artificial intelligence, namely the problem of building algorithms that can learn continually without corrupting previously learned information,” he said. “The brain manifestly achieves this, and this work is a step in the direction of finding algorithms that can do the same.”
Reference:
Michael E. Rule; Timothy O’Leary. ‘Self-healing codes: How stable neural populations can track continually reconfiguring neural representations.’ PNAS (2022). DOI: 10.1073/pnas.2106692119